The know-how utilized by Facebook, Google and Amazon to show spoken language into textual content, acknowledge faces and goal promoting may assist docs fight one of many deadliest killers in American hospitals.
Clostridium difficile, a lethal bacterium unfold by bodily contact with objects or contaminated folks, thrives in hospitals, inflicting 453,000 instances a yr and 29,000 deaths within the United States, in keeping with a 2015 study within the New England Journal of Medicine. Traditional strategies corresponding to monitoring hygiene and warning indicators usually fail to cease the illness.
But what if it have been attainable to systematically goal these most weak to C-diff? Erica Shenoy, an infectious-disease specialist at Massachusetts General Hospital, and Jenna Wiens, a pc scientist and assistant professor of engineering on the University of Michigan, did simply that once they created an algorithm to predict a affected person’s danger of creating a C-diff an infection, or CDI. Using sufferers’ very important indicators and different well being data, this methodology — nonetheless in an experimental section — is one thing each researchers need to see built-in into hospital routines.
The CDI algorithm — primarily based on a type of synthetic intelligence known as machine studying — is at the forefront of a technological wave beginning to hit the U.S. well being care trade. After years of experimentation, machine studying’s predictive powers are well-established, and it’s poised to maneuver from labs to broad real-world purposes, mentioned Zeeshan Syed, who directs Stanford University’s Clinical Inference and Algorithms Program.
“The implications of machine learning are profound,” Syed mentioned. “Yet it additionally guarantees to be an unpredictable, disruptive drive — more likely to alter the way in which medical selections are made and put some folks out of labor.
Machine studying (ML) depends on synthetic neural networks that roughly mimic the way in which animal brains be taught.
As a fox maps new terrain, as an example, responding to smells, sights and noises, it regularly adapts and refines its habits to maximise the percentages of discovering its subsequent meal. Neural networks map digital terrains of ones and zeroes. A machine studying algorithm programmed to establish photos of espresso cups would possibly examine photographs of random objects towards a database of espresso cup photos; by analyzing extra photos, it systematically learns the options to make a optimistic ID extra rapidly and precisely.
Email Sign-Up
Subscribe to KHN’s free Morning Briefing.
Shenoy and Wiens’ CDI algorithm analyzed a knowledge set from 374,000 inpatient admissions to Massachusetts General Hospital and the University of Michigan Health System, in search of connections between instances of CDI and the circumstances behind them.
The data contained over four,000 distinct variables. “We have knowledge pertaining to every little thing from lab outcomes to what mattress they’re in, to who’s within the mattress subsequent to them and whether or not they’re contaminated. We included all medicines, labs and diagnoses. And we extracted this each day,” Wiens mentioned. “You can imagine, as the patient moves around the hospital, risk evolves over time, and we wanted to capture that.”
As it repeatedly analyzes this knowledge, the ML course of extracts warning indicators of illness that docs might miss — constellations of signs, circumstances and particulars of medical historical past most certainly to lead to an infection at any level within the hospital keep.
Such algorithms, now commonplace in web commerce, finance and self-driving automobiles, are comparatively untested in drugs and well being care. In the U.S., the transition from written to digital well being data has been sluggish, and the format and high quality of the info nonetheless differ by well being system — and typically right down to the medical follow degree — creating obstacles for pc scientists.
But different traits are proving inexorable: Computing energy has grown exponentially whereas getting cheaper. Once, making a machine studying algorithm required networks of mainframe computer systems; now it may be carried out on a laptop computer.
Radiology and pathology will expertise the adjustments first, consultants say. Machine studying packages will most simply deal with analyzing photos. X-rays and MRI, PET and CT scans are, in any case, plenty of information. By crunching the info contained in 1000’s of current scan photos together with the diagnoses docs have made out of them, algorithms can distill the collective data of the medical institution in days or hours. This permits them to duplicate or surpass the accuracy of any single physician.
Machine studying algorithms can now reliably diagnose skin cancers (from images) and lung cancer, and predict the risk of seizures.
Google analysis scientist Lily Peng, a doctor, led a workforce that developed a machine studying algorithm to diagnose a affected person’s risk of diabetic retinopathy from a retinal scan. DR, a standard facet impact of diabetes, can result in blindness if left untreated. The worldwide rise in diabetes charges has turned DR into a worldwide well being drawback, with the variety of instances expected to rise from 126.6 million in 2011 to 191 million by 2030 — a rise of practically 51 p.c. Its presence is indicated by more and more muddy-looking scan photos.
Peng’s workforce gathered 128,000 retinal scans from hospitals in India and the U.S. and assembled a workforce of 54 ophthalmologists to grade them on a 5-point scale for indicators of the illness. Multiple docs reviewed every picture to common out particular person variations of interpretation.
Once “trained” on an preliminary knowledge set with the diagnoses, the algorithm was examined on one other set of information — and there it barely exceeded the collective efficiency of the ophthalmologists.
Now Peng is engaged on making use of this instrument in India, the place a continual scarcity of ophthalmologists means DR usually goes undiagnosed and untreated till it’s too late to save lots of a affected person’s imaginative and prescient. (This can be an issue within the U.S., the place 38 p.c of grownup diabetes sufferers don’t get the advisable annual eye verify for the illness, in keeping with the Centers for Disease Control.)
A bunch of Indian hospitals is now testing the algorithm. Ordinarily, a scan is completed, and a affected person might wait days for outcomes after a specialist — if accessible — reads the picture. The algorithm, through software program operating on hospital computer systems, makes the outcomes accessible instantly and a affected person may be referred to remedy.
Last yr, the Food and Drug Administration accepted the primary medical machine studying algorithm for business use by the San Francisco firm Arterys. Its algorithm, “DeepVentricle,” performs in 30 seconds a job docs sometimes do by hand — drawing the contours of ventricles from a number of MRI scans of the center muscle in movement, as a way to calculate the amount of blood passing by way of. That takes a mean of 45 minutes. “It’s automating something that is important — and tedious,” mentioned Carla Leibowitz, Arterys’ head of technique and advertising and marketing.
If adopted on a broad scale, such applied sciences may save a number of money and time. But such change is disruptive.
“The fact that we have identified potential ways to gut out costs is good news. The problem is the people who get gutted are not going to like it — so there will be resistance,” mentioned Eric Topol, director of the Scripps Translational Science Institute. “It undercuts how radiologists do their work. Their primary work is reading scans — what happens when they don’t have to do that?”
The shift might not put quite a lot of docs out of labor, mentioned Topol, who co-authored a piece in JAMA exploring the difficulty. Rather, it’s going to doubtless push them to search out new methods to use their experience. They might deal with more difficult diagnoses the place algorithms proceed to fall brief, as an example, or work together extra with sufferers.
Beyond this frontier, algorithms can present a extra exact prognosis for the course of a illness — doubtlessly reshaping remedy of progressive illnesses or addressing the uncertainties in end-of-life care. They can anticipate fast-moving infections like CDI and continual illnesses corresponding to coronary heart failure.
As the U.S. inhabitants ages, coronary heart failure can be a rising burden on the well being system and on households.
“It’s the most expensive single disease as a category because of the extreme disability it causes and the high demand for care it imposes, if not managed really tightly,” mentioned Walter “Buzz“ Stewart, vice chairman and chief analysis officer at Sutter Health, a well being system in Northern California. “If we may predict who was going to get it, maybe we may start to intervene a lot earlier, possibly a yr or two years sooner than when it normally occurs — after we admit a affected person to the hospital after a cardiac occasion or crash.”
Stewart has collaborated on a number of research aiming to deal with that drawback. One, done with Georgia Tech computer scientist Jimeng Sun, predicts whether or not a affected person will develop coronary heart failure inside six months, primarily based on 12 to 18 months of outpatient medical data.
These instruments, Stewart mentioned, are resulting in the “mass customization of health care.” Once algorithms can anticipate incipient phases of circumstances like coronary heart failure, docs can be higher capable of supply remedies tailor-made to the affected person’s circumstances.
Despite its scientific promise, machine studying in drugs stays terra incognita in some ways. It provides a brand new voice — the voice of the machine — to key medical selections, as an example. Doctors and sufferers could also be sluggish to just accept that. Adding to potential doubts, machine studying is usually a black field: Data go in, and solutions come out, however it’s usually unclear why sure patterns in a affected person’s knowledge level, say, to an rising illness. Even the scientists who program neural networks usually don’t perceive how they attain their conclusions.
“It’s going to make a big difference in how decisions are made — things will become much more data-driven than they used to be,” mentioned John Guttag, a professor of pc science at MIT. Doctors will depend on these more and more complicated instruments to make selections, he mentioned, and “have no idea how they work.” And, in some instances, will probably be onerous to determine why dangerous recommendation was given.
And whereas well being knowledge are proliferating, the amount, high quality and format differ by establishment, and that impacts what the algorithms “learn.”
“That is a huge issue with modeling and electronic health records,” Sun mentioned. “Because the data are not curated for research purposes. They are collected as a byproduct of care in day-to-day operations, and utilized mainly for billing and reimbursement purposes. The data is very, very noisy.”
This additionally implies that knowledge could also be inconsistent, even in a person affected person’s data. More essential, one measurement doesn’t match all: An algorithm developed with knowledge from one hospital or well being system might not work properly for an additional. “So you need models for different institutions, and the models become quite fragile, you might put it,” Sun mentioned. He is engaged on a National Institutes of Health grant finding out learn how to develop algorithms that can work throughout establishments.
And the tide of obtainable medical knowledge continues to rise, tantalizing scientists. “Think about all the data we are collecting right now,” Wiens mentioned. “Electronic health records. Hospitalizations. At outpatient centers. At home. We are starting to collect lots of data on personal monitors. These data are valuable in ways we can’t yet know.”
Related Topics Health Industry Hospitals Patient Safety
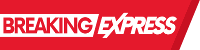